By Holli White, MPS, Director of Health System Partnerships, HDAI
Background
* Rose Taylor, an 81-year-old female patient, suffered from an Acute Myocardial Infarction (AMI) and was hospitalized for seven days. Upon release, she had no follow-up primary care or cardiology appointment scheduled and passed away 14 days after her hospital discharge. Could her death have been prevented? Perhaps.
Within the realm of cardiac care, the 30-day all-cause mortality rate is a pivotal measure, offering crucial insights into patient outcomes and enabling healthcare institutions to benchmark their performance against national standards. Hospitals who have a culture of continuous improvement are hyper-focused on inpatient mortality but reducing post-discharge mortality requires a different mindset that stretches outside the hospital walls.
Rose’s story is an example of patients whose post-discharge mortality may have been preventable. Even leading healthcare systems with historically low inpatient mortality rates can take steps to address suboptimal 30-day mortality rates post-discharge.
Different approaches must be taken to identify high-risk patients, enabling clinicians to efficiently and effectively leverage existing care pathways and strategies that are aimed at reducing early mortality risks and elevating patient care standards.
Risk Predictions to Enhance Patient Management
Accurate, real-time, enterprise-wide risk prediction models that are embedded in the medical record can inform patient triage and treatment decisions, reduce randomness, and improve targeting of patients in the top quintile of mortality risk. While these risk prediction models do not dictate specific interventions, they play a crucial role by flagging patients who require heightened attention during admission, discharge, and follow-up, enabling timely, targeted interventions that can lower post-discharge mortality rates.
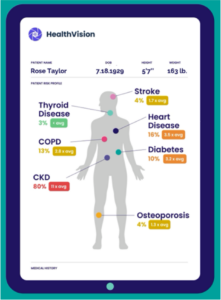
Models and Methodology
Health Data Analytics Institute (HDAI) has developed over 400 predictive models that can be used for patients who are admitted, recently discharged, or in the community (not admitted or recently discharged). The models rely on demographic data (age, sex), diagnoses codes (ICD-10), and event codes (CPT) to calculate likelihood of the defined outcome. Here the specific focus is predicting and identifying patients like Rose, who are at higher risk for 30-day all-cause mortality or readmission.
HDAI’s model methodology and performance has been featured in peer-reviewed publications over the last decade, with the latest conducted with the Center on Outcomes Research at Cleveland Clinic and published in the Journal of Anesthesiology in December 2022 and February 2023.
HDAI’s EMR-embedded or SaaS platform, HealthVisionTM, serves as the Intelligent Health Management System and displays these predictive models and other crucial information to providers and healthcare leaders. HealthVision is transforming care across health systems and value-based care organizations and is made up of three key components:
- The Intelligent Health Record, showcasing the Patient Spotlight, is an easy to digest health profile and risk prediction tool. The Patient Spotlight helps clinicians make sense of the increasingly unwieldy and unmanageable health data in their EMRs (Electronic Medical Record) in real-time. See the sample Patient Spotlight view.
- The Intelligent Workflow Solution, which includes smart cohorts and rosters that align scarce clinical, documentation, and administrative resources with the patients who will benefit the most.
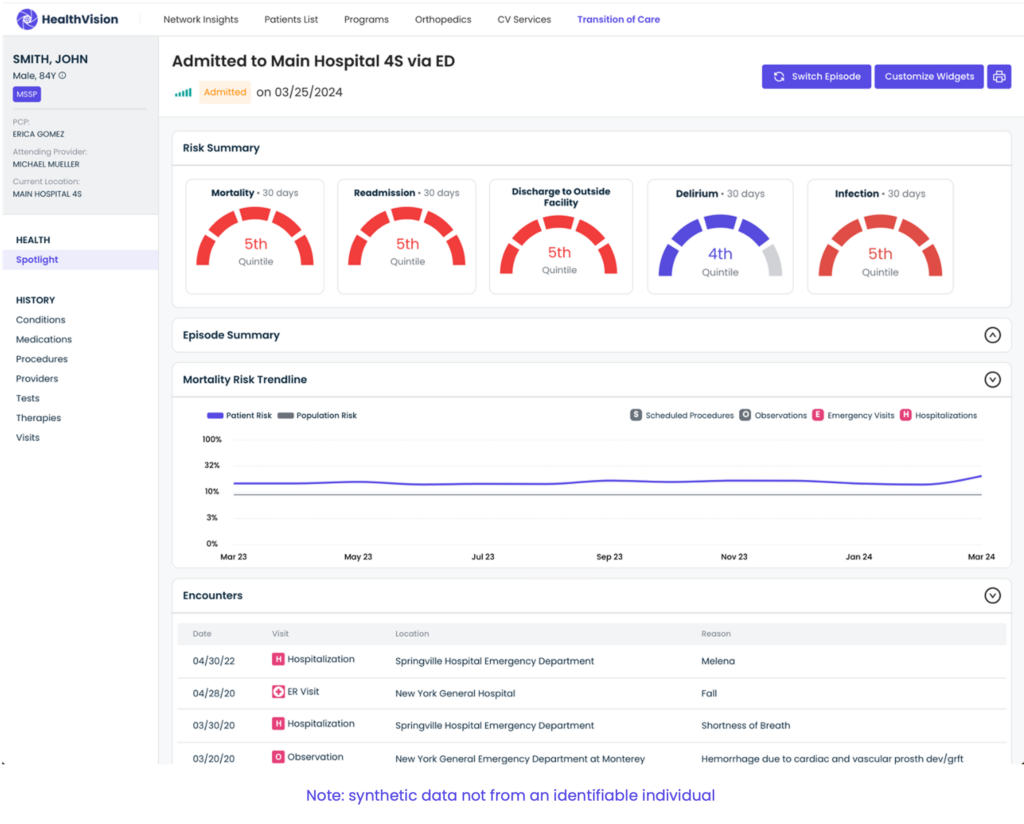
- The Intelligent Analytics Solution, matching a population to others outside of an organization with the same baseline risk results to fairly assess performance of providers or post-acute networks. These precise comparisons are unlike traditional benchmarks in that they take into consideration illness burden at the patient level and suggest actions that can improve outcomes for each risk group.
The 30-Day Mortality Risk Score Model for Cardiac Patients
- PTCA with or without stent placement
- Insertion; revision; replacement; removal of cardiac pacemaker or cardioverter/defibrillator
- Heart valves: repair and replacement (includes TAVI)
- Acute myocardial infarction
- Heart failure and shock
The model identifies the top 20th percentile of patients at risk for all-cause mortality within the next 30 days. By pinpointing such high-risk individuals, clinicians can implement targeted evidence-based interventions, including personalized medication regimens, 7-day follow-ups, and timely specialist consultations to mitigate risks, enhance patient safety, and optimize resource utilization.In retrospect, Rose was identified as high-risk for mortality and readmission within the next 30 days from her discharge. If she had been flagged during her hospital stay and provided with additional services aimed at her specific risk drivers, her outcome post-discharge could have been quite different.
Ensuring Success through Actionability
A critical factor for success is that the data must be actionable for the clinical team. The risk prediction models, such as the 30-day mortality risk score model, aim to identify patients at the highest risk of all-cause mortality within the next 30 days. These high-risk patients can be flagged using the risk scores provided by the model. The risk scores can be displayed in different formats, such as absolute risk, relative risk, percentile risk, or risk summary (categorized as High, Medium, or Low), to support different use cases and clinician preferences.
For example, in AMI patients like Rose, specific risk factors may include previous myocardial infarctions, comorbidities such as diabetes or hypertension, and indicators of cardiac function such as ejection fraction. HealthVision, integrated into the electronic health record system, can then utilize these identified risk factors to provide actionable insights for the clinical team. For example, when a patient with an AMI or HF specific DRG is admitted, updated risk prediction scores can automatically calculate and display the patient’s risk score based on their demographic data, medical history, and new diagnoses pulled from clinical notes and problem lists. This risk score can serve as a prompt for clinicians to assess the patient’s condition more closely and consider appropriate interventions.
Based on the identified risk factors, tailored care pathways can be designed. For Rose and other AMI patients, the first step is to set up a follow-up appointment within 7 days of discharge. Subsequent interventions could include initiation of guideline-directed medical therapy (e.g., aspirin, beta-blockers, ACE inhibitors), referral for cardiac rehabilitation, and education on lifestyle modifications (e.g., smoking cessation, dietary changes).
In conclusion, by leveraging advanced analytics, personalized insights, and real-time guidance, clinicians can make more informed decisions, optimize treatment outcomes, and ultimately improve the overall quality and efficiency of healthcare delivery. Saving lives, like Rose, with data-driven approaches and personalized care.
* This is an illustrative case and not based on an actual patient, though it is representative of many individuals like her.
Contact us!
If you would like to discuss your work in the healthcare space and learn more about HDAI, please reach out to [email protected]. We would love to speak with you.
SHARE