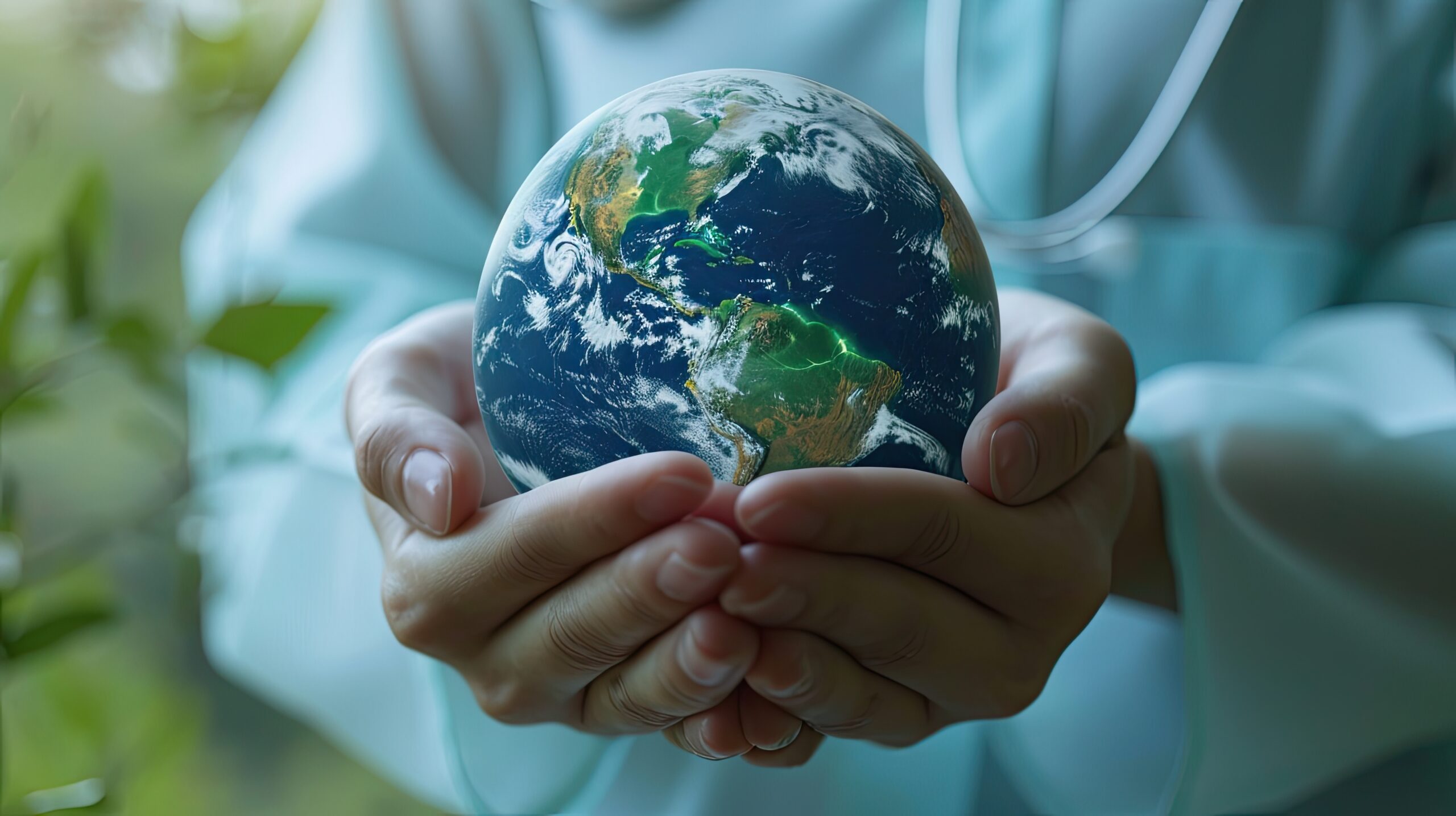
By Nassib Chamoun
Background
As the inevitable transition to an “intelligent health system” accelerates, the development, implementation, and deployment of AI-powered solutions is moving at speeds uncharacteristic of healthcare. Leveraging intelligence in all aspects of healthcare management and operations is becoming essential in helping to address many of the industry’s most pressing challenges, including clinician burnout, increased complexity of care, strained resources, operational inefficiencies, and EHR-inflicted productivity drag.
The Promise
Large Language Models (LLMs) provide powerful new capabilities that can uniquely support many transformational solutions in healthcare. With LLMs, the ability to unlock the value of unstructured data is at our fingertips like never before. Proponents of Generative AI promise unprecedented improvements in productivity, personalization, and reductions in clinician burnout. The belief is that as data rapidly changes, spanning large corpuses of tokenized text, images, and audio, the LLMs will adapt, leveraging their immensely deep and complex weights to provide thoughtful, ethical, and accurate responses across a variety of use cases in near-real time.
Reality Check
However, beyond meeting performance and responsible healthcare AI requirements, little consideration has been given to the energy consumption and related carbon emissions associated with the computational burden of the training, response generation, and fine-tuning of these AI models to support healthcare use cases. Perhaps the most overlooked factor in delivering AI at scale in healthcare is the “inference rate” which is driven by frequency at which these inputs are changing and the rate at which the model outputs must be updated. For AI to enable enterprise-wide healthcare use cases, including generation of clinical documentation, extraction of structured data from medical records, risk stratification, diagnostics, and utilization, cost, and operational predictions, it must efficiently thrive in a high inference rate environment.
The Cost
Recently, The New York Times reported, “by 2027 AI servers could use between 85 to 134 terawatt hours annually. That’s similar to the annual energy use of Argentina or Sweden¹.” A major contributing factor to this projection is the widespread adoption of energy-intensive technologies such as LLMs and Generative AI models, including GPT models. With healthcare generating more than 30% of all data globally, the deployment of AI at scale across the sector is likely to drive a disproportionate share of the forecasted energy use by AI servers in the next 5 years. A recent eye-opening study by researchers at AI startup Hugging Face and Carnegie Mellon University found that using large generative models to create outputs was far more energy intensive than using smaller AI models tailored for specific tasks². This highlights the importance of selecting for each use case an AI architecture optimized for the appropriate tradeoffs between performance and cost.
Fit to Purpose
The resulting costs from broad adoption of LLMs in healthcare could be far greater than anticipated and are likely to further strain extremely challenged healthcare budgets. Of course, such expenses would be justified if the impact on productivity or patient outcomes far exceeded the investment. STAT News summarized several papers that highlighted significant shortcoming of notes or assessments generated by LLMs including more time spent by clinicians reading AI-generated responses³. In addition, a study evaluating an experimental institution-wide deployment of GPT-4 for research and operational exploration at Dana-Farber Cancer Institute raised many questions including significant cost concerns even within the limited scope of their deployment⁴.
Roberta Schwartz, Executive Vice President and Chief Innovation Officer at Houston Methodist, made it clear at a recent conference that AI in healthcare must always rely on “a human in the loop” and that it cannot distract or further burden the user. In my view today, the best “human in the loop” for LLMs are experienced healthcare AI developers supported by clinical experts who can leverage the power of LLMs to rapidly develop, train and optimize targeted algorithms using more efficient and understandable AI tools that meet the performance, cost, and transparency requirements necessary for healthcare.
HDAI's Experience
Beyond published data, many who have used LLMs extensively in healthcare know that while some of the output is remarkable, much of it is also very concerning. This aligns with Health Data Analytics Institute (HDAI)‘s experience where in collaboration with Houston Methodist, we analyzed millions of physician notes with GPT-3.5/4.0 to extract diagnostic codes. In parallel, we processed the same data with HDAI’s deterministic NLP algorithm based on methodologies we have been using for many years. The results, which also included human review of thousands of notes, led us to discard more than 90% of the codes generated by GPT due to redundancies within ICD families or total hallucinations that were completely off base. Furthermore, the cost and time required to process the notes was nearly 1,000 times greater for GPT when compared to our targeted NLP solution. On the other very positive side, GPT was able to identify some complex patterns that were missed by traditional models. Ultimately, we adopted a hybrid approach that leverages the strengths of each technology to deliver a performant, cost-effective and high-throughput solution that can be deployed at-scale for our intended use case.
Path Forward
Many of the recently published studies and our direct experience in using LLMs make it difficult to see LLMs as a primary solution for use cases with high inference frequency anytime soon due to a wide range of challenges⁵⁶⁷. Beyond the cost and performance issues, we also need to be cognizant of responsible healthcare AI requirements, including consistency and reproducibility of results, transparency, and explainability. Nonetheless, today we can leverage LLMs and GPTs for training cheaper, faster, and task-specific AI models or run them in parallel in a more cost-effective hybrid framework that can deliver urgently needed capabilities. Several collaborative communities are forming to guide others along this path. VALID AI out of University of California, Davis and Ashish Atreja, MD, MPH are already deeply focused on these issues including the development of targeted Small Language Models (SLMs).
The "Intelligent Health System"
As Harry P. Pappas and Paul Frisch, PhD state in their new book, The Rise of the Intelligent Health System, “…an Intelligent Health System is an entity that leverages data and AI to create strategic advantages through the efficient provision of health and medical services across all touchpoints, experiences, and channels⁸.”
Looking ahead, the major challenge for US healthcare is the need to focus on the paradigm shift required to successfully transition to an environment where embedded intelligence becomes an integral part of every operational and clinical workflow. Market leaders will take advantage of the rapid cycles of innovation by tech companies to transform the way they deliver care. Health systems must invest in the AI literacy of their workforce by exposing them to solutions and technologies that enable frontline workers to experiment, learn, innovate, and reinvent their work environment. I look forward to the day in the not-so-distant future when widespread, responsible use of AI will significantly reduce the burden on clinicians, enhance care-team productivity, and deliver better, cost-effective outcomes for patients.
Contact us!
If you would like to discuss your work in the healthcare space and learn more about HDAI, please reach out to [email protected]. We would love to speak with you.
References
“A.I. Could Soon Need as Much Electricity as an Entire Country” https://www.nytimes.com/2023/10/10/climate/ai-could-soon-need-as-much-electricity-as-an-entire-country.html
“Power Hungry Processing: Watts Driving the Cost of AI Deployment?” https://arxiv.org/pdf/2311.16863
“Generative AI is supposed to save doctors from burnout. New data show it needs more training.” https://www.statnews.com/2024/04/25/health-ai-large-language-models-clinical-documentation
“GPT-4 in a Cancer Center: Institute-Wide Deployment Challenges and Lessons Learned” https://ai.nejm.org/doi/full/10.1056/AIcs2300191
“AI-Generated Clinical Summaries Require More than Accuracy” https://jamanetwork.com/journals/jama/article-abstract/2814609
“AI-Generated Draft Replies Integrated Into Health Records and Physicians’ Electronic Communication” https://jamanetwork.com/journals/jamanetworkopen/fullarticle/2817615?resultClick=1
“Large Language Models are Poor Medical Coders – Benchmarking of Medical Code Querying” https://ai.nejm.org/doi/full/10.1056/AIdbp2300040
“The Rise of the Intelligent Health System” by Paul H. Frisch and Harry P. Pappas https://www.routledge.com/The-Rise-of-the-Intelligent-Health-System/Pappas-Frisch/p/book/9780367769345