By Carola Endicott
‘Tis the season for looking ahead to the new year. Will every category of health tech be able to support 10-20 thriving companies? And will 2022 be the year that AI delivers on its promise to help clinicians? No and yes. Please read on.
In a recent STAT, Katie Palmer noted “Among health tech companies that went public in 2021 — both through SPAC mergers and traditional IPOs — stock prices have fallen an average of roughly 45% since the close of their first day of trading.” And in MedCity News, Elise Reuter asked “what happens when the music stops?” Our industry is flooded with investment dollars fueling startups whose goal is to innovate and disrupt as they create a new normal. No doubt we’ll see some spectacular successes; the health care industry desperately needs that. But it’s unrealistic to expect that we’ll have 10 or 20 thriving companies in every segment. It’s highly likely we’ll see one or two category-defining companies that win the lion’s share of revenue and customers. So, in 2022, we think we’ll continue to see a thinning of the herd. You can’t sell vision forever – companies will increasingly need to show rigorous proof that they can deliver tangible results. As we saw in 2021, many will come up short of expectations.
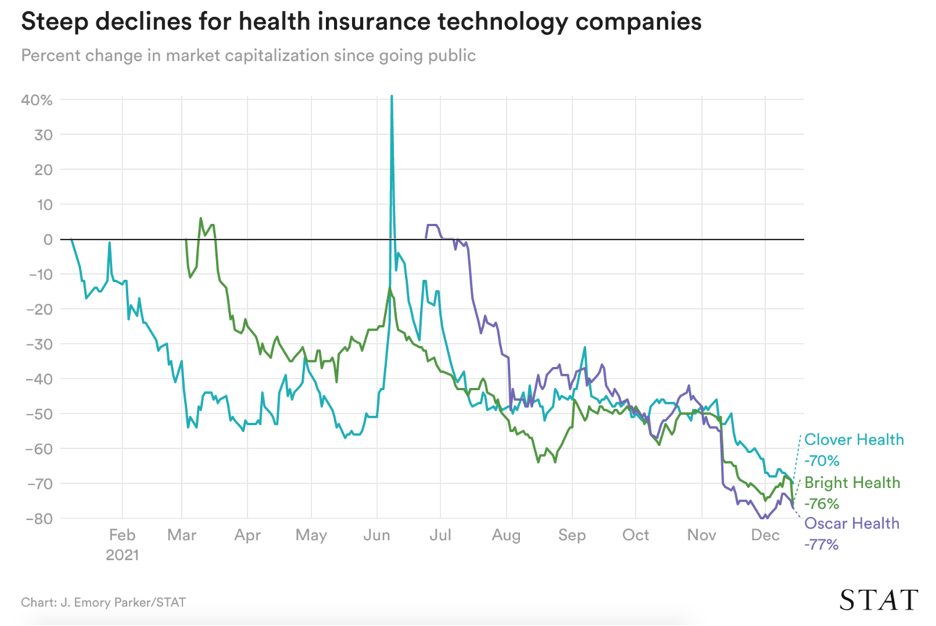
And what about AI? After all these years, there is still a mix of enthusiasm and skepticism about its potential to improve healthcare. Clinicians, looking for relief from data overload and time scarcity, will start to learn how to incorporate AI-assists into their diagnostic workups for complex patients, as noted in a recent JAMA article by Julia Adler-Millstein. This is the year that early advocates using AI in controlled settings will lead the way to broader adoption at the point of care. As machine learning moves into the clinic, clinicians and the public will become increasingly aware of how predictive models should be used and the pitfalls to avoid. This will lead to a high-stakes debate about the strengths and especially the limitations of machine learning applications as some algorithms fail with possibly dire consequences while other solutions fueled by ML and deep clinical involvement, will be shining examples of what is possible.
The Dynamic Diagnostic Refinement Process (from the link above)
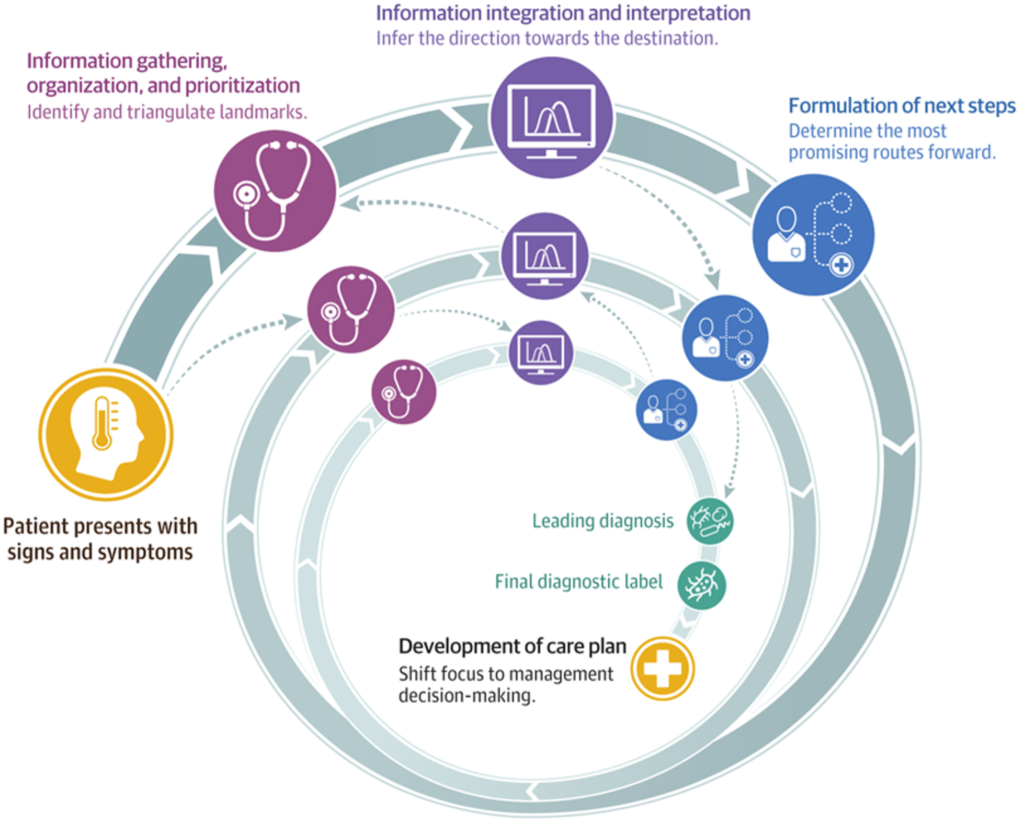